Data Privacy in Autonomous Vehicles
I. Introduction: What is an ADAS Dataset?
An Advanced Driver Assistance Systems (ADAS) dataset is a collection of data used in autonomous driving systems to aid in detection and decision-making.
The essence of an Advanced Driver Assistance Systems (ADAS) dataset is quite akin to teaching a newbie how to drive. As you mentor the young driver, you guide them through diverse scenarios, from navigating a bustling city street to handling the tranquillity of a countryside road. They learn to interpret and respond to different situations and make decisions based on these experiences. The role of an ADAS dataset mirrors this process. It is a vast compendium of real-life driving situations, recorded using sophisticated sensors like cameras, LiDAR, and RADAR. This critical data forms the backbone of the ADAS, instructing it on how to comprehend and react appropriately to a variety of driving conditions.
The ADAS dataset is integral to the future of autonomous driving, serving as the heart of the system that shapes secure and efficient navigation. The ADAS architecture involves the meticulous integration of sensor input, intelligent data processing, and the strategic orchestration of responses.
Imagine the complexity and richness of data points collated from a variety of sensors like LiDAR, RADAR, cameras, and GNSS. This enormous data stream is processed in real-time by advanced algorithms, making quick yet informed decisions akin to the split-second decision-making of a human driver. Through each unique road scenario, ADAS continues to learn and evolve, thereby demonstrating the power of these tracking datasets collected.
Progression in ADAS also signifies a roadmap towards total vehicle autonomy. As we move from Level 0 to Level 5 of automation, the driver's role gradually diminishes, transitioning more control to the vehicle itself. Although full autonomy (Level 5) hasn't been achieved yet, rapid advancements in ADAS technology suggest a future where the vehicle holds greater autonomy isn't too distant.
In essence, the ADAS dataset is at the crux of a system aimed at revolutionizing road safety. It embodies the collaborative effort of advanced technology and human creativity to enhance road safety and drive experiences. As we see an increase in ADAS-equipped vehicles on the roads, it's clear we're not merely experiencing a technological shift; we're participating in a global movement towards safer and more advanced driving experiences.
Understanding the ADAS dataset is key to this journey towards autonomous driving. In the further sections, we'll delve deeper into the specifics of sensor technologies, data interpretation methods, and the various levels of ADAS automation. This exploration will offer valuable insights into the future of autonomous driving, furthering our understanding of ADAS.
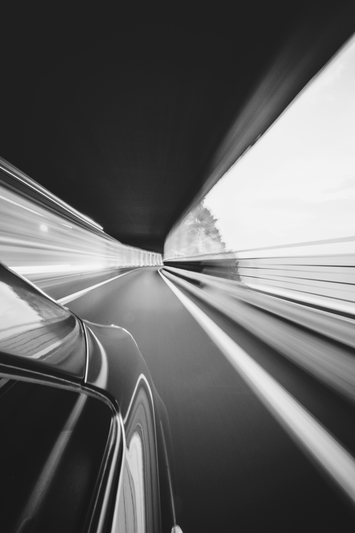
II. Understanding the Importance of GDPR Compliance for ADAS Datasets in Autonomous Vehicles
Navigating the intricacies of GDPR compliance in the realm of Advanced Driver Assistance Systems (ADAS) is no small task. As these technologies proliferate and semi-autonomous vehicles become more common, the amount of data collected skyrockets. This surge in data collection inevitably triggers privacy concerns. For instance, faces and license plates, often captured in ADAS images and videos, fall under the definition of personal data as per Article 4 of the GDPR.
The accepted legal perspective posits that private entities, such as automotive companies, are permitted to process personal data only when there isn't a reasonable alternative. In most scenarios revolving around research and development, recognizing specific individuals or vehicles isn't a necessity. Therefore, in the absence of any other option, data minimization through anonymization should be applied (our previous articles on What is the Right to be Forgotten in GDPR? and What is Data Anonymization? provide a comprehensive overview of these aspects of GDPR). This approach not only helps avoid potential legal issues but also minimizes the risk of penalties, public backlash, and erosion of customer trust that can occur if personal data is processed without a compelling reason or a feasible anonymizing alternative.
Furthermore, when an automotive company has plans to store or share data for future projects, or with third-party companies, similar considerations must be taken into account. Anonymization's importance can't be overstated — in fact, the process of complete and irreversible anonymization can make the application of GDPR regulations irrelevant.
Even for universities and research centers, despite certain GDPR provisions granting some leeway for data processing for public interest, scientific research, or statistical purposes, such privileges become moot if complete anonymization is feasible. It further underlines the paramount importance and benefits of thorough and irreversible anonymization in fulfilling GDPR obligations and maintaining data privacy.
III. How do Autonomous Cars Collect Data?
Data is the lifeblood of Autonomous Vehicles. Data collection is primarily done through various sensors that the vehicle is equipped with.
Peeling back the layers of the ADAS technology, we uncover a multifaceted mesh of sensors working relentlessly. These sensory systems, much like human senses, collect data incessantly, providing the essential information for the advanced functionalities of ADAS. Adaptive cruise control, traffic warnings, lane departure and centering, and collision avoidance are just a handful of the features this data powers.
Key sensory components that comprise this robust system include:
- RADAR (Radio Detection and Ranging): This sensor plays an instrumental role in preventing collisions and identifying pedestrians and cyclists. It complements vision-based camera-sensing systems by being able to detect objects at distances reaching up to 300 meters.
- LiDAR (Light Detection and Ranging): This sensor, an offshoot of RADAR, leverages lasers for real-time object detection and distance mapping. High-grade sensors are equipped with up to 128 lasers to create highly accurate 3D point clouds.
- V2X (Vehicle to Everything): This feature promotes seamless communication between the vehicle and any entity that may affect or be affected by it. This includes infrastructures, networks, other vehicles, pedestrians, and devices.
- GNSS (Global Navigation Satellite System): This advanced navigation system provides centimeter-level accuracy, a requisite for achieving true autonomy.
- Camera: Multiple cameras work in concert to provide a comprehensive view of the surroundings. They play a vital role in traffic sign recognition, reading road markings, and recognizing obstacles.
After the data is harvested through these sensors, it progresses through an intricate data enrichment process, which can be broken down into several stages:
- Data Collection: The initial capture of data from various sensors such as RADAR, LiDAR, cameras, GPS/GNSS, and SONAR.
- Data Preparation: At this stage, the collected data is reviewed and labeled, and metadata is added. This enrichment process prepares the data for the subsequent stages.
- Test Suite Creation: This involves the construction of models, scenarios, simulations, and anticipated reactions.
- Validation: Both the hardware and software are tested using the test suites created in the previous step.
- Analysis: Once testing is complete, the results are examined, tests are managed, and reports are created to document the findings.
- Archiving: This step involves long-term retention of data, allowing for future use and quick restoration when necessary.
- Usage: Finally, the enriched data is put to work for design and development, for training ADAS algorithms, and for the creation of modules.
However, the collected data often contain personal information. For instance, visual data collected by cameras can include images of faces and license plates. Hence, measures like Face Blurring, License Plate Blurring, and Car Plate Blurring must be taken to ensure data anonymization and GDPR compliance.
It's important to remember that all data collected should align with the principles of GDPR, as discussed in our blog post on Privacy by Design and Default. This principle requires that data protection measures are integrated into the design of data collection systems.

IV. Key Challenges in Ensuring GDPR Compliance in ADAS Datasets for Vehicle Bus Data
Incorporating GDPR Compliance into ADAS datasets is fraught with challenges. To start with, the volume and complexity of data collected by autonomous vehicles is massive, making it difficult to effectively monitor and manage. With various sensor inputs including cameras, LiDAR, RADAR, and GNSS, there's an abundance of data points that potentially contain personally identifiable information (PII).
This PII could include facial features, license plates, and even the geographical location of individuals – all caught in the purview of an autonomous vehicle's sensors. Therefore, GDPR compliance becomes a significant challenge when you need to constantly blur faces and license plates, anonymize data, and secure it from potential breaches.
Moreover, the multinational nature of automotive companies further complicates the application of GDPR. The regulation is an EU law, but many companies operate globally. Ensuring GDPR Compliance for Autonomous Vehicles across different jurisdictions can be quite a task. GDPR's impact on ADAS datasets is considerable, and understanding these GDPR Regulations for Autonomous Cars is paramount for any company in the industry.
V. What are the Privacy Issues with Self-Driving Cars?
The era of autonomous vehicles comes with significant privacy concerns. These vehicles' inherent nature of continuous connectivity and the Advanced Driver Assistance Systems (ADAS) datasets they gather underline several privacy challenges.
The ADAS datasets act as a treasure trove of personal data, which includes, but is not limited to, images of faces, license plates, and highly sensitive location data. The misuse potential of this information is enormous, especially if it inadvertently lands in unscrupulous hands. Although safeguards like face and license plate blurring are in place, the risk to privacy is indelible, considering the granular nature of data collected.
Data breaches form another significant threat. Should hackers compromise autonomous vehicles, they could exploit the personal data within or manipulate the vehicle's systems, leading to dangerous consequences. As a result, data security in self-driving cars is of paramount importance, necessitating stringent safeguards.
However, addressing privacy issues in autonomous vehicles requires an intricate understanding of the General Data Protection Regulation (GDPR), especially when processing personal data. Consent typically serves as a legal basis for processing personal data, and for sensitive data, it's an exception to the general prohibition (Art 7, Art 9, GDPR).
This issue has been emphasized by Maria Cristina Gaeta who is a scholar at the Suor Orsola Benincasa University of Naples [See: Gaeta M.C. (2017), The issue of data protection in the Internet of Things with particular regard to self-driving cars, DIRITTO MERCATO TECNOLOGIA. pp. 1-20, ISSN: 2239-7442].
Consent, though, poses unique challenges in the context of autonomous vehicles. For instance, in an emergency at lower automation levels (level 3), constant solicitation of consent could jeopardize safety. In scenarios involving Vehicle-to-Infrastructure (V2I) and Vehicle-to-Vehicle (V2V) communication, instantaneous data exchange is necessary, leaving no room for obtaining user consent.
Further complicating the situation is the fact that autonomous vehicles gather data not only about the driver but also about passengers and possibly individuals outside the vehicle. Traditional consent models fail to accommodate such instances, indicating the imperative necessity of personal data processing in this context.
This complexity necessitates the extensive application of data protection regulations to highly automated cars. It calls for sector-specific legislation for autonomous vehicles to successfully navigate the path towards full automation.
According to GDPR, express consent is one of the lawful bases for processing personal data, with specific exceptions in cases of personal data categories, profiling, and personal data transfers to third countries or international organisations (recital 32, Art 9, Art 22, Art 49 para 1, lett a, GDPR).
Recital 32 of the GDPR recognises any explicit, positive act indicating user consent for personal data processing, such as online consent, as lawful. However, there exist certain actions associated more closely with implied consent, particularly in electronic means, which do not fit neatly within the 'positive act' definition. Moreover, in specific scenarios as highlighted in the Proposal for a Regulation on Privacy and Electronic Communications, consent is not a requisite, thereby highlighting the complexity of the matter.
In conclusion, privacy issues are paramount in the self-driving cars domain, necessitating careful scrutiny and application of regulations such as the GDPR. To ensure the safe, efficient, and ethical operation of these vehicles, privacy challenges need to be addressed head-on with robust and sector-specific legislative frameworks.
(Reference: Gaeta, M.C. (2017). The issue of data protection in the Internet of Things with particular regard to self-driving cars. DIRITTO MERCATO TECNOLOGIA, pp. 1-20, ISSN: 2239-7442.)
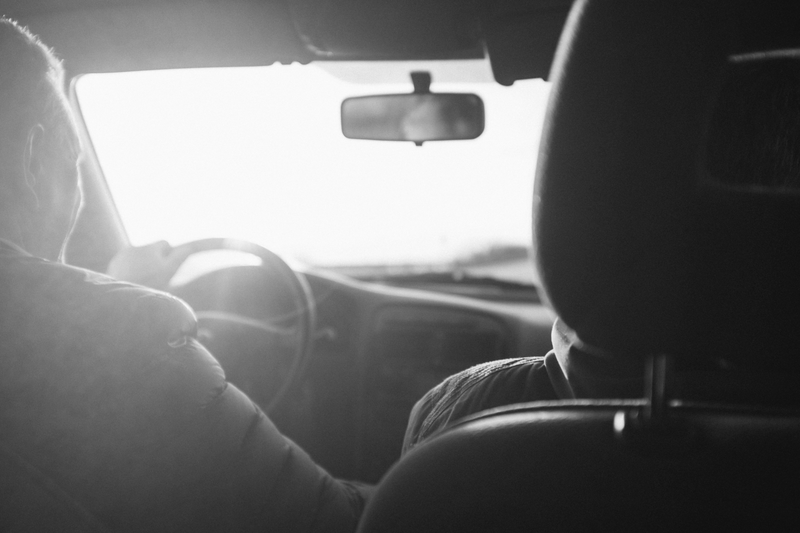
VI. Strategies for Maintaining GDPR Compliance in ADAS Datasets for Autonomous Driving
Despite the challenges, several strategies can be adopted to maintain GDPR compliance in ADAS datasets. Privacy should be at the heart of data collection and processing activities. This is part of a broader principle known as 'Privacy by Design and Default,' which we've covered in a separate blog post.
One of the foremost strategies is to minimize data collection. Only collect what is necessary for the ADAS to function correctly. Additionally, robust anonymization techniques, such as face blurring, car plate blurring, and other methods of data masking, can help protect personal information.
Regular audits and compliance checks are crucial for maintaining GDPR compliance in an ongoing manner. A systematic approach to record-keeping can also help, as discussed in our blog entry on Record of Processing Activities.
Implementing these strategies can greatly enhance GDPR compliance and help avoid the severe penalties associated with non-compliance.
VII. How do You Check if a tracking dataset collected is GDPR-Compliant?
Determining if an automotive company adheres to GDPR rules can be a complex task due to the nature of ADAS and its expansive dataset. However, several indicators can help assess their compliance status. The first place to look is their privacy policy. GDPR demands transparency from companies about how they collect, process, and store data. A comprehensive and clear privacy policy usually suggests a commitment to GDPR compliance.
Next, look at the company's data protection infrastructure. Robust anonymization processes, like face blurring, license plate blurring, and general data anonymization, are good signs. Check also if they have a system in place for responding to data requests and breaches, an essential part of GDPR compliance.
The role of a Data Protection Officer (DPO) is crucial in maintaining GDPR Compliance for Driver Assistance Systems. An appointed DPO suggests a company's earnest effort in GDPR compliance.
Finally, the proof is in the pudding. If a company has a record of GDPR violations or data breaches, it's a red flag.
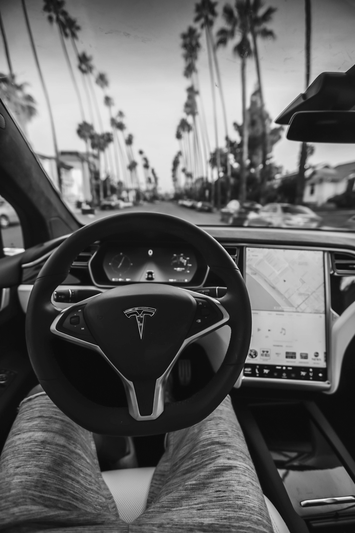
VIII. How Long Can You Store ADAS Data from sensor suite under GDPR?
GDPR rules on data storage are clear — personal data should only be kept as long as necessary for the purpose it was collected. However, defining 'necessary' in the context of ADAS datasets can be challenging.
The continuous operation of autonomous vehicles and the need for data in improving their performance and safety could justify prolonged data retention. Yet, companies must balance this against GDPR regulations and the privacy rights of individuals.
The principle of data minimization, as we discussed in our blog post about data anonymization, is a good guide here. It advises to collect only the data that is necessary, use it only for its intended purpose, and keep it only as long as required.
IX. Ethical Challenges for AI and ADAS Datasets
While GDPR provides a legal framework for handling ADAS datasets, ethical considerations also play a significant role. Autonomous vehicles, powered by AI, pose unique ethical challenges that society must grapple with.
The primary concern is privacy. Despite best efforts to blur faces and anonymize data, the constant data collection by autonomous vehicles raises profound privacy issues.
Another ethical question revolves around decision-making in life-threatening situations. In the event of a potential accident, how should an autonomous vehicle react? How it responds will be dictated by the AI, which in turn is trained using ADAS datasets. This raises further questions about how such datasets are created, processed, and used.
As outlined in a recent article on Tooploox, some of the key ethical issues in ADAS datasets include:
- The first issue is the problem of reason-effect in ADAS datasets. These systems often make decisions based on patterns they find in the data. However, they can sometimes mistake correlation for causation. For example, if an ADAS system is trained primarily on data collected during daylight hours, it may not perform as effectively at night. This could lead to potentially unsafe outcomes.
- Another ethical concern arises from the inherent inhumanity of artificial neural networks. Despite their sophistication, these systems lack human intuition and context awareness. This can lead to unpredictable responses to unique or unforeseen road conditions. The 'Black Box' problem – the inability to fully understand how a machine learning model arrives at its decisions – also contributes to this challenge.
- Bias is another critical ethical issue, as it can creep into datasets in subtle ways. For example, if an ADAS dataset is predominantly comprised of data from highways, the system may not be as effective on rural roads. This can lead to unintentional bias and may affect the safety and reliability of autonomous vehicles.
- Creating large, diverse, and legally compliant ADAS datasets is also fraught with ethical difficulties. GDPR, which restricts the use of personal or sensitive data, may limit the types of data that can be included in these datasets. This could affect the system's ability to learn from and adapt to a wide array of conditions and situations.
- Imbalances in gender and demographic representation within ADAS datasets can also lead to bias. For instance, if the majority of data comes from male drivers, the system may not fully understand or anticipate the driving behaviors of female drivers.
- The issue of accurately representing reality in ADAS datasets is another important concern. Ideally, these datasets should reflect a wide range of driving conditions, road types, and driver behaviors. However, readily available data may not provide a complete picture of real-world scenarios, which can limit the effectiveness of ADAS systems.
- To address these ethical challenges, several AI ethics policies and governance initiatives have been introduced. These aim to guide the ethical development of AI technologies, including ADAS. Despite these complexities, one thing is clear: the need for responsible creation and use of ADAS datasets is paramount. Only by carefully navigating these ethical challenges can we fully harness the potential of ADAS technologies, ensuring they are safe, fair, and effective for all road users.

IX. Conclusion
As we navigate these ethical waters, it's critical that developers and regulators engage in ongoing dialogue to ensure that ADAS technologies respect not only legal requirements but ethical ones too. Balancing technological progress with privacy and ethics will be key in our journey towards a future filled with autonomous vehicles.
Ensuring GDPR Compliance in ADAS Datasets for Autonomous Vehicles is a multi-faceted and complex process. However, with a thorough understanding of GDPR principles, and with the right strategies in place, it is achievable. Privacy Challenges in Autonomous Vehicles are certainly not insurmountable.
From a legal perspective, the most prudent and effective approach to ensuring GDPR compliance within ADAS datasets is to completely purge them of personal data. This process, often referred to as 'data sanitization', involves systematically removing or anonymizing data that could potentially infringe upon privacy regulations.
To aid in this endeavor, there are specialized software solutions available that can automate the data sanitization process on an industrial scale. One such solution is Gallio PRO, an AI-powered software that can be run on a server or a desktop. The distinct advantage of this solution is its ability to operate entirely on-premise. This stands in stark contrast to cloud-based alternatives, as on-premise solutions inherently offer greater assurance that data processing activities align with stringent GDPR requirements.
Legal Disclaimer: The information provided in this article is for general informational purposes only and does not constitute legal advice. We are not legal practitioners, and as such, this article should not be used as a substitute for professional legal advice. In each specific case, we strongly recommend consulting with a qualified lawyer to address your unique legal concerns and ensure compliance with applicable laws and regulations.